Benchmarking Results: Google Gemini Pro vs. OpenAI GPT4 for Healthcare Applications
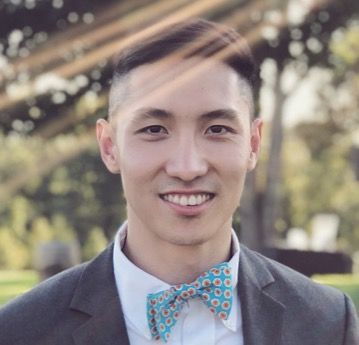
The potential of AI applications in the healthcare sector is immense, over $70B was invested into AI and AI-enabled startups in 2023. The proliferation of generative AI models like OpenAI's GPT-4, Anthropic's Claude, Meta's LLaMA-2, and most recently Google's Gemini has given rise to thousands of new AI applications, thanks to low barriers to entry and ease of implementation.
According to McKinsey, one-third of organizations in its Global Survey have already adopted AI within one or more departments, with plans to accelerate investments owing to advancements in generative AI. Although the transformational potential of AI, particularly in healthcare, is undeniable, recent meta-analyses found that only 10-20% of commercially available AI-enabled applications have been externally validated or undergone academic-level reviews.
We believe the lack of rigor did not stem from malice or ill intent, but rather from the absence of standards and a validation framework. To put it in layman's terms: when it comes to validating models, most founders and executives don't know where to start.
Objective: Testing Computer-assisted Diagnosis with AI
The goal of this report is not to establish a standard framework for validation across the healthcare sector, but rather to provide a preliminary insight into the capabilities of Google's Gemini Pro in handling semi-complex tasks in healthcare. In this instance, we are evaluating Gemini's medical domain knowledge through two tests: (1) physical and (2) dermatological conditions. We are eager to test the capabilities of Gemini Ultra; if you have a connection at Google, please contact us.
Although it is the most controversial use case, we are particularly interested in benchmarking AI's capability for clinical diagnosis. This interest is driven by the recent consensus that clinical diagnosis is the holy grail application for unlocking productivity in population health.
Testing Method
Models used
OpenAI's GPT-4-32K and Google's Gemini Pro (Gemini Ultra is unavailable for commercial access at the time of testing). Disclaimer: we have not sponsored or received any monetary compensation from either Google or OpenAI.
Prompt
Beginning in early 2022, we at Mira started experimenting with applied AI, actively incorporating generative AI into various aspects of our business. Additionally, we launched a care companion feature named Beacon. We are applying the prompt engineering techniques acquired during the development of Beacon to conduct these tests.
Design
Test 1: Physical Conditions – In this test, our objective is to evaluate both Gemini and GPT-4 using 45 clinical vignettes, mirroring a prior study conducted by Semigran HL, Linder JA, Gidengil C, and Mehrotra A titled "Evaluation of Symptom Checkers for Self-Diagnosis and Triage," originally published in 2015 and updated 2023.
Test 2: Dermatological Conditions – For this test, we assess Gemini's performance on the 20 most common skin conditions, supplemented by 20 additional novel cases sourced publicly from Dermnet. In total, 40 vignettes are utilized for this evaluation.
Credit
Shridhar Gambhir, our Product Manager and John Murcia, our engineer also took part in constructing and completing the report.
Baseline
Physical conditions
We are using the accuracy metrics provided in Mehrotra A. et al.'s 2023 report as a baseline for our evaluations. Here, accuracy is defined as the ability to "list the correct diagnosis within the top three options."
Sample | Year | Accuracy |
---|---|---|
23 symptom checkers | 2015 | 51% |
Physicians (n=234 physicians who solved at least 1 vignette, 90% were trained in internal medicine and 52% were fellows or residents) | 2016 | 85% |
ChatGPT3-3.5 | 2022 | 82% |
ChatGPT4 | 2022 | 87% |
Dermatological conditions
We are using the sensitivity and accuracy measures from a 2020 meta-analysis by Abhishek De, Aarti Sarda, Sachi Gupta, and Sudip Das as baselines for our tests.
Study Design | Research | Results |
---|---|---|
Deep convolutional neural network (AI) vs 13 board-certified dermatologists (MDs): 14 diagnoses malignant and benign conditions | Fujisawa et al. 2019 | 92.4% AI vs. 85.3% MDs (accuracy) |
Deep learning algorithm to classify the clinical images of 12 skin diseases including melanoma | Han et al. 2018 | 85.1% (sensitivity) |
804 biopsy-proven dermoscopic images of melanoma and nevi were presented to dermatologists (MDs) and convolutional neural network (AI) | Brinker et al. 2019 | 82.3% AI vs. 67.2% MDs (sensitivity) |
Results
When analyzing both Gemini Pro and GPT4-32K in the physical condition tests, it was found that both models performed with an equivalent sensitivity of 93.33%. Both were successful at identifying the correct diagnosis in 42 out of 45 clinical vignettes. However, Gemini Pro was found to have slightly higher confidence levels, with an average of 71.40% confidence compared to GPT4's 65.24%. This suggests that while both models demonstrated similar diagnostic accuracy, Gemini Pro showed a marginal superiority in its degree of certainty when drawing conclusions.
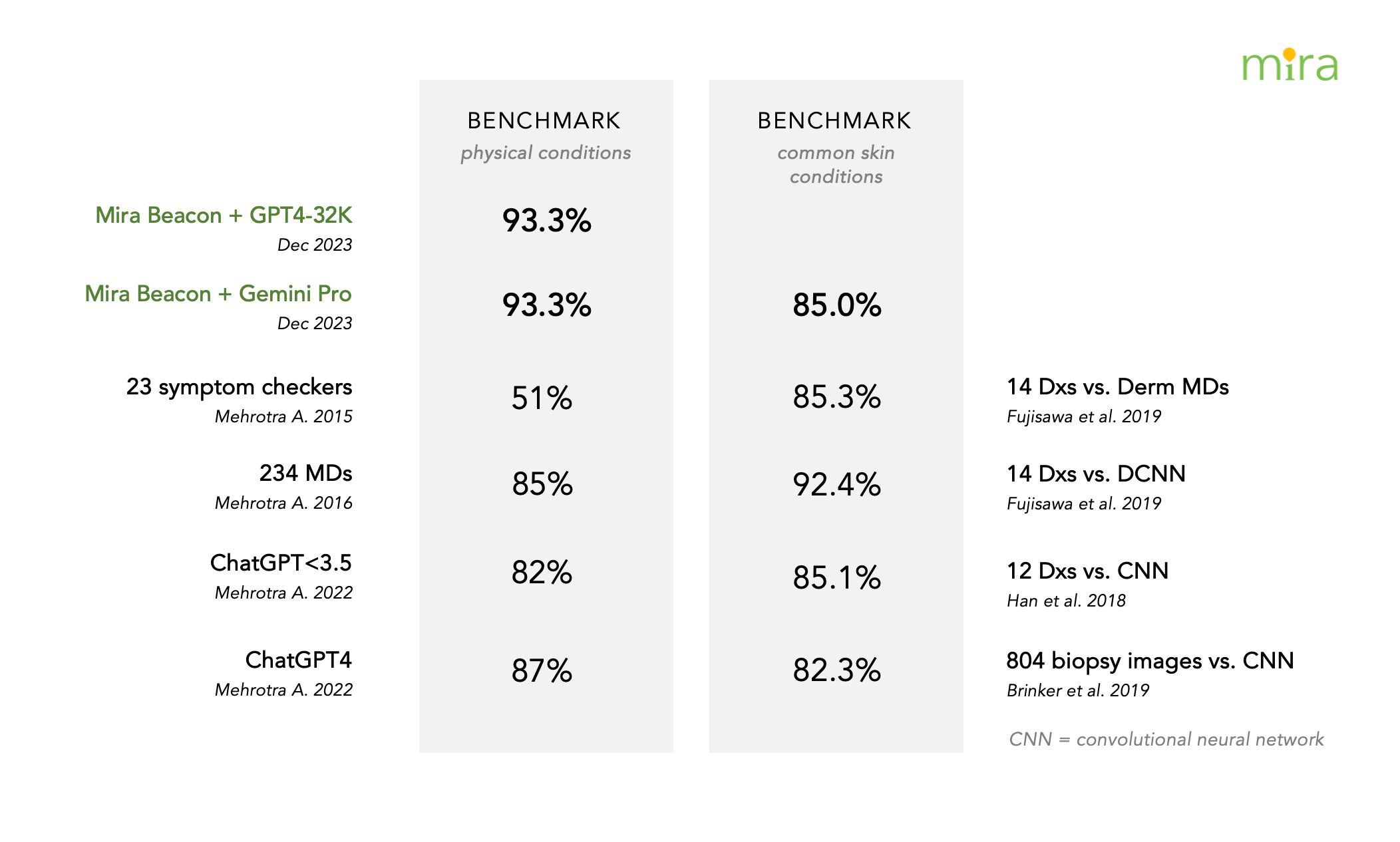
Overall | Total Vignettes | Correct | Sensitivity | Confidence % |
---|---|---|---|---|
Gemini Pro | 45 | 42 | 93.33% | 71.40% |
GPT4-32K | 45 | 42 | 93.33% | 65.24% |
Emergent | Total | Correct | Sensitivity | Confidence % |
---|---|---|---|---|
Gemini Pro | 15 | 15 | 100.00% | 70.00% |
GPT4-32K | 15 | 15 | 100.00% | 64.33% |
Non-emergent | Total | Correct | Sensitivity | Confidence % |
---|---|---|---|---|
Gemini Pro | 15 | 13 | 86.67% | 72.14% |
GPT4-32K | 15 | 14 | 93.33% | 62.50% |
Self-care Appropriate | Total | Correct | Sensitivity | Confidence % |
---|---|---|---|---|
Gemini Pro | 15 | 14 | 93.33% | 72.14% |
GPT4-32K | 15 | 13 | 86.67% | 69.23% |
In the dermatological condition tests, there was a divergence between Gemini Pro's efficiency in recognizing novel skin cases and the top 20 common skin conditions.
For novel skin cases, Gemini Pro accurately identified the condition in 60.00% of cases, with 9 out of 20 cases correctly diagnosed. It also managed to recognize similar conditions in 15% of the cases, rendering an overall effectiveness of 60.00% and an average confidence level of 55%.
When it came to the analysis of the most common skin conditions, Gemini Pro clearly excelled, scoring an accuracy rate of 85.00% with 17 out of 20 cases correctly diagnosed, showing a solid performance in recognizing prevalent dermatological conditions.
Novel Skin Cases | Total | Exact | Similar | Sensitivity | Confidence % |
---|---|---|---|---|---|
Gemini Pro | 20 | 9 | 3 | 60.00% | 55% |
Top 20 Common Skin Conditions | Total | Correct | Sensitivity | Confidence % |
---|---|---|---|---|
Gemini Pro | 20 | 17 | 85.00% | 69% |
Conclusion
Compared to previous research, both Gemini Pro and GPT4-32K tested with Mira's Beacon showed excellent diagnostic capabilities for physical conditions, with an overall sensitivity of 93.33%. This is significantly higher than the 51% accuracy reported for symptom checkers in the 2015 Mehrotra A. et. al study, and marginally higher than the 85% accuracy showcased by physicians in 2016. This result outperforms the performance of ChatGPT3-3.5 at 82% and is close to ChatGPT4's previous diagnostic accuracy score of 87% reported in 2022.
For the novel dermatological condition tests, Gemini Pro, with an average diagnostic accuracy of 60.00%, somewhat lags behind the benchmark set by deep learning algorithms in Han et al's 2018 study, which recorded a sensitivity of 85.10%. This suggests that, while promising, the model's ability to recognize complex skin cases is still in nascent stages compared to existing AI technology.
However, when it came to recognizing the top 20 most common skin conditions, Gemini Pro showed an impressive accuracy level of 85.00%, nearly matching the previous AI benchmark of 85.3% set by Fujisawa et al in 2019 and significantly outperforming the earlier reported physician's sensitivity of 67.2% in Brinker et al's 2019 study.
In conclusion, AI models like Gemini Pro and GPT4-32K mark a significant milestone in healthcare, demonstrating a transformative potential in diagnosis, especially when compared to physicians' traditional diagnostic methods. However, it's also clear that further refinements are needed for these models to consistently achieve or exceed the highest benchmarks set by their AI predecessors.
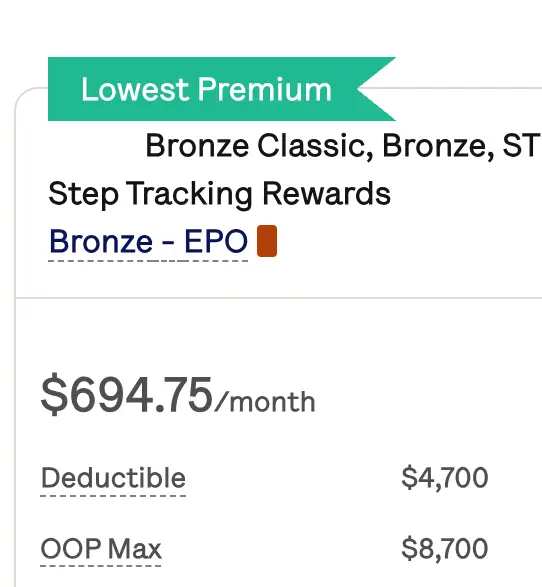
Can't afford traditional health insurance? Get Mira - Healthcare you can afford.
Join 36,000 people and get Mira. Plans start at $45/mo. No paperwork. No wait period.
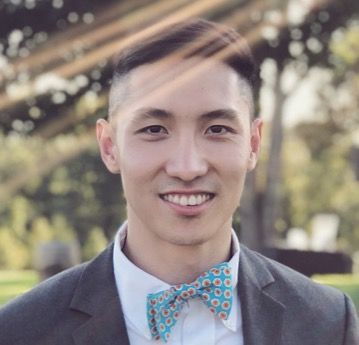
Khang T. Vuong received his Master of Healthcare Administration from the Milken Institute School of Public Health at the George Washington University. He was named Forbes Healthcare 2021 30 under 30. Vuong spoke at Stanford Medicine X, HIMSS conference, and served as a Fellow at the Bon Secours Health System.